Data analysis has become a useful skill for post-graduate careers and a necessary tool for social research.
To celebrate this year’s Love Data Week, we would like to take you on a field trip to the world of computational social research. The field embraces the wealth of information and opportunities in big data, leverages the power of computational approaches, and emphasizes the scientific rigor in social research.
If you are curious about the field, or a newcomer, we would like to recommend three books below to you. These books are accessible. They help readers to generate ideas of studying society and human behaviors and offer a toolbox of methodological and technical instruments.
Bit by Bit – Social Research in the Digital Age
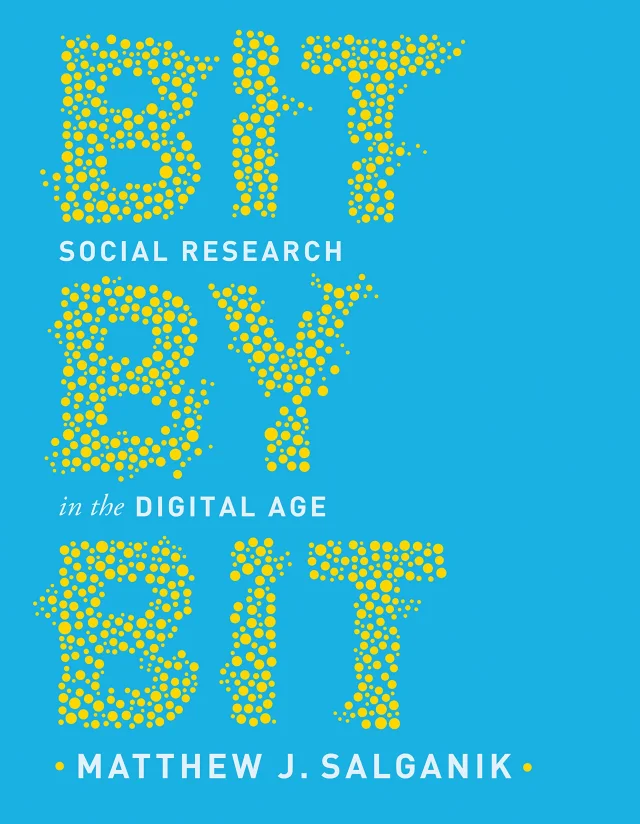
What does social research look like when it enters the digital match? The book uses cases from disciplines of social sciences and other areas such as biology and law to explain how to design social research.
The author has worked in government (at the US Census Bureau) and in the technology industry (at Microsoft Research), and is currently Professor of Sociology at Princeton.
You may read this book online from its website https://www.bitbybitbook.com/en/1st-ed/preface/.
Quantitative Social Science – An Introduction
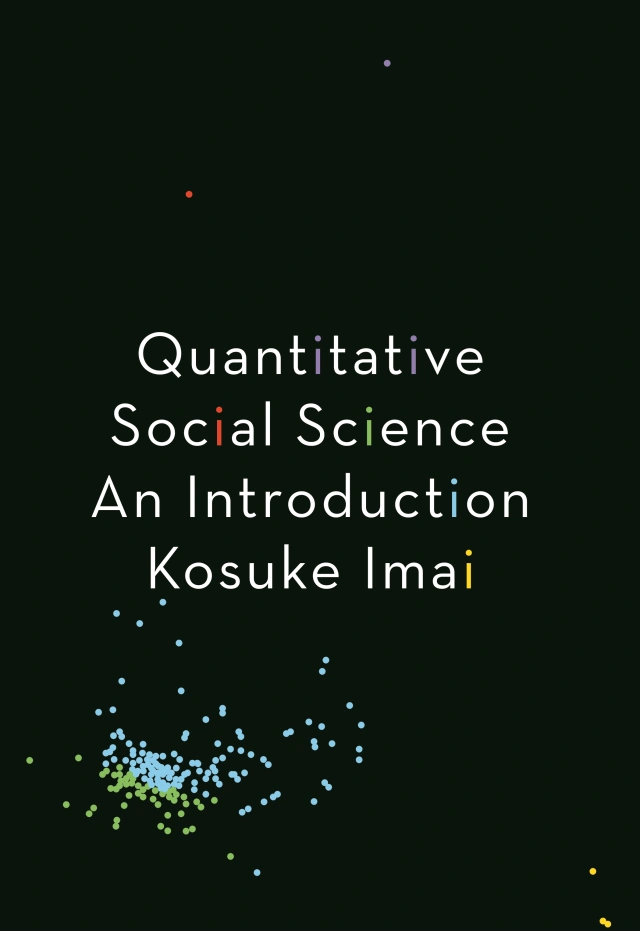
The book combines three essential components of data analysis: topics of interest, methodological concepts, and computer programming. Each chapter couples a concept in data analysis (e.g. causality, measurement, prediction, and probability) with an introduction of a core construct in the R language, using many data sets from published research. It also covers the practical and applied aspects of data analysis, including visualization, and exploratory analysis with textual, network, and spatial data.
The author is Professor of Government and of Statistics at Harvard University. He specializes in the development of statistical methods and machine learning algorithms and their applications to social science research.
The data sets and R scripts for all of the chapters of this book can be found at the author’s GitHub repository https://github.com/kosukeimai/qss.
Text as Data – A New Framework for Machine Learning and the Social Sciences
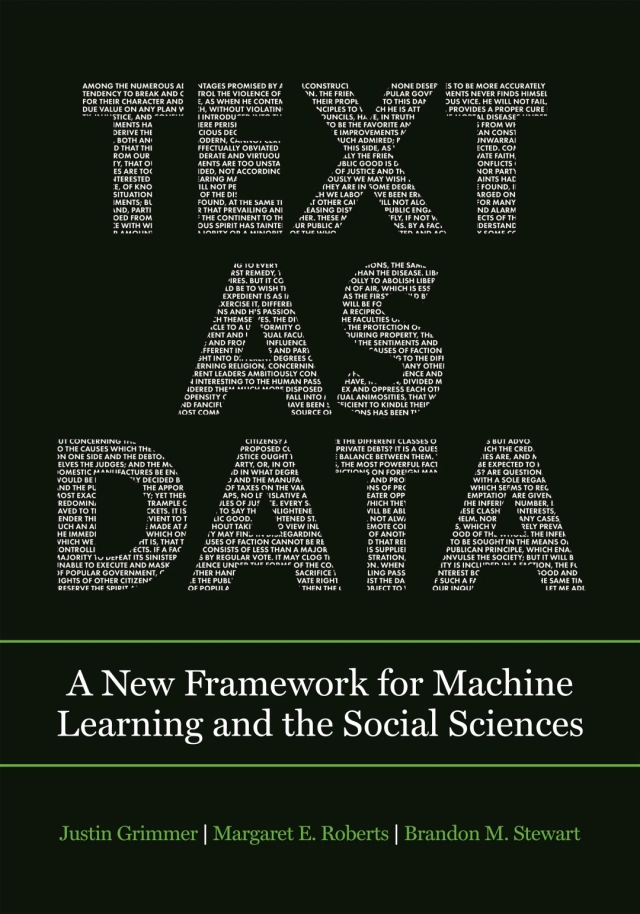
The last book introduces how text can be included in every stage of the research process–discovery, measurement, prediction, and causal inference.
It describes the frequently used models in text analysis, focusing on how the abundance of text and new statistical methods facilitate inferences rather than the technical details of using text analytics to perform a specific task.
The authors are professors of political science and sociology at Stanford University, University of California San Diego, and Princeton University.
Related to this book, the course materials for Text as Data can be found in this GitHub repository: https://github.com/justingrimmer/tad_19.
Contact
Yun Dai (yun.dai@nyu.edu)
Data Services @ NYU Shanghai Library shanghai.hosting.nyu.edu/data